4 Use Cases of Predictive Analytics in Retail
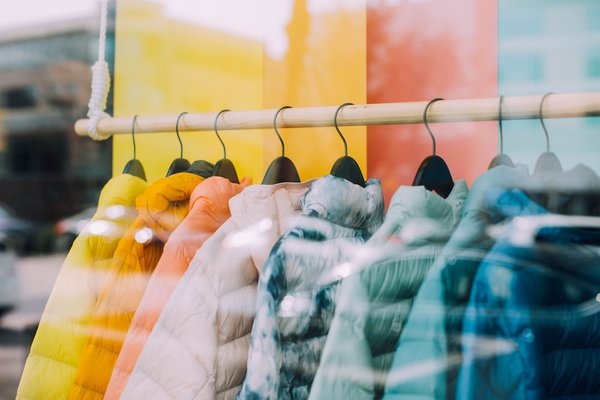
Retailers and the manufacturers who supply them have long had massive amounts of data at their disposal. But what to make of that data was always in question.
We live in a world where we generate a truly staggering amount of data every year, and this figure is increasing exponentially. The research firm IDC estimates that by 2025, there will be 175 Zettabytes (ZB) of data in the global datasphere. For context, that number stood at 33 ZB as recently as 2018. Data serves as fuel for one of the hottest subfields of artificial intelligence (AI) in the digital age, predictive analytics.
Predictive analytics utilizes data to gain valuable insights and make predictions about how things will behave in the future. Armed with this information, retailers become empowered to create better products, reach the right customers, boost conversion rates, and stay ahead of the competition. With this in mind, here are the top four use cases for predictive analytics in the retail sector.
Assortment Planning
At its core, assortment planning is about putting the right products in front of the right customers. This can be optimizing a store shelf or the product pages on an e-commerce site. However, determining the right mix of products and their quantities is a big challenge for any retailer, large or small. It forces businesses to ask questions like:
- How much weight do you give to insights gained from customer surveys? (Are consumers the best judge of what they really want?)
- How do you know how much stock of a new product line to buy when there's no sale history to back it up?
- What products should go where? What should customers see first? What should they see when they’re at the checkout?
- Which assortment of products will maximize profits the most?
Assortment planning used to be done on pen or paper and eventually graduated to spreadsheets, but today, thriving companies are using predictive analytics.
Benefits of Predictive Analytics in Assortment Planning
AI-based assortment planning software uses variables like demographics (age, sex, marital status, occupation), regional differences (culture, climate, local preferences), and store location (city, tourist hotspot, countryside) to make optimal merchandise decisions. It also takes into account market trends, shopping patterns, past performance, and customer sentiment.
The More Data, the Better
Predictive analytics works because it's a comprehensive and science-based approach that reveals opportunities you might otherwise miss or helps avoid costly mistakes. Relying on just a few data sources to inform business decision-making can lead to potentially catastrophic mistakes. For example, in 2009, Walmart decided to declutter its store based on feedback from customer surveys. The message was clear - customers felt the aisles were too distracting and wanted less clutter. But did they? Being a responsive business, Walmart decided to cut merchandise by around 15%. Customer satisfaction scores skyrocketed, but there was a problem. Sales plummeted, and the company lost around $1.85 billion. With predictive analytics, Walmart could have avoided this expensive blunder altogether.
Inventory Management
Poorly managed inventory can lead to frustrated customers, steep productivity losses, and lackluster margins. Luckily, predictive analytics takes care of a lot of the heavy lifting today. Predictive analytics software can ensure companies always have the right amount of a product, avoiding stock-outs or overstocks. It can also:
- Suggest changes to the stock based on customer perception towards certain vendors or materials. For example, sustainability and ethical buying are fast becoming a number one priority for consumers today. When a brand gets a reputation for using environmentally questionable products, the fallout can be significant.
- Reduce stock risks. Companies that rely on just a few vendors can struggle with inventory management when unforeseen events harm the supply chain.
Benefits of Predictive Analytics For Inventory Management
A well-managed inventory pipe supports increased cash flow by reducing obsolescence and the amount of cash tied up in goods. It also improves customer service and brand loyalty by ensuring customers can find what they are looking for, or get their orders delivered on time.
Sending Promotions to Customers at the Right Time
Timing can be the difference between landing a sale or having a customer float off to a competitor. For example, did you know you're more likely to respond to a marketing email on a Monday or Tuesday than any other day? In the digital age, predictive analytics software serves to boost the success of tried and tested customer management strategies like loyalty programs or customer relationship management systems. The data collected by these systems, which ranges from the company's website to communication channels and social media, helps companies learn more about their target audience.
Benefits of Predictive Analytics For Time-Targeted Promotions
- Reengaging customers - Most businesses have loyal customers (brand promoters), unhappy customers (brand detractors), and those in-between. Customers that fall in the middle often don't feel strongly enough about a company's products to actively browse for new products. However, if they are presented with a new product that aligns with their wants or needs, they will engage.
- Customer segmentation - Splitting your customers into distinct segments can drive higher conversion rates and boost sales. It can also help identify the right time to approach certain customers. For example, a mother with school-aged children will be more likely to engage with promotions on back-to-school items in the late summer, before kids go back to school.
- Reducing customer churn - Predictive analytics software can determine how likely a customer is to migrate to a competitor. Armed with this information, businesses can offer a promotion at the right time and avoid losing valuable customers.
Price and Promotion Optimization
Getting pricing right is critical to the success of any business, but it's not always straightforward. Beyond just how items are priced, how this price is communicated can dramatically impact how customers engage with the product.
First, the retailer or etailer has to establish their overarching price strategy. Walmart revolutionized the industry with everyday low pricing (“EDLP”) schemes, offering consistently discounted prices and only very limited temporary discounts called “rollbacks.” At the height of uncertainty during Covid in 2020, Tesco reportedly moved to a similar EDLP model. Meanwhile, alternative “high-low” pricing schemes support better gross margins for most products, allowing for deep price discounts on certain high demand “loss leader” goods that reinforce to shoppers they’re getting a great deal. The what, when, and how much pricing questions are the never-ending details retailers obsess upon. AI models enable them to crunch much larger numbers more rapidly, opening new opportunities to excite consumers and beat the competition.
Price and promotion simulation algorithms allow companies to try different strategies in a controlled and safe environment to avoid costly mistakes further down the line. For example, it helps businesses decide whether customers will respond better to 'dollar-off promotions like "$10 off X product" or a percentage-based offer like "20% off". It can also highlight which products are best for cart-based promotions, where the offer is applied to the shopping cart rather than on individual products, for example, "15% off all orders over $150".
Where to Go Next
In days past, tried and true analog methods for the four areas discussed above were good enough to compete. “Planograms” would lay out the products to go on the shelf on a sheet of paper, and most retailers would mock up a sample shelf in a warehouse to see how it looked. Inventory was managed with costly enterprise tools that were essentially well-organized linked spreadsheets. Promotion and price optimization were done with simple statistical methods like regression analysis. However, AI models for predictive analysis have rapidly outpaced that “tried and true.” Massive amounts of data can now be collected, classified, and analyzed for deeper insights. Companies that embrace predictive analytics technology for day-to-day decisions will quickly leave the laggards behind.
A number of Plug and Play startups leverage AI and machine learning to make predictive analytics more accessible to retailers and CPG companies alike. For example, Algo improves sales and operations planning with data-driven workflows and deep supply chain and inventory analysis. Simporter offers analytics that de-risk new product development, automating R&D discovery at the ideation stage and predicting sales before market entry by analyzing billions of consumer data points. Retina.ai provides accurate customer lifetime value metrics early in the customer journey.
Get to know more of the startups by checking out both ourSupply Chain andBrand & Retail platforms and attending our summits. The data is out there. It’s critically important to know what to make of it.